Data science is revolutionizing the path to sustainable construction, transforming how we design, build, and operate our built environment. By harnessing the power of advanced analytics and machine learning, construction professionals can now predict energy consumption patterns, optimize resource utilization, and minimize environmental impact with unprecedented precision.
The convergence of data science and sustainability represents a critical turning point for the construction industry. Real-time sensors, IoT devices, and sophisticated modeling algorithms are enabling projects to achieve up to 30% reduction in energy consumption and 25% decrease in material waste. These technological advances aren’t just environmental wins – they translate directly to cost savings and improved project outcomes.
For construction leaders and decision-makers, the integration of data-driven sustainability solutions has become non-negotiable. From BIM-enabled carbon footprint analysis to AI-powered equipment optimization, these tools provide the quantitative insights needed to make informed decisions about sustainable building practices. The challenge now lies not in whether to adopt these technologies, but in how to implement them effectively to maximize both environmental and economic benefits.
This strategic alignment of data science capabilities with sustainability goals is creating new opportunities for innovation in construction, setting new standards for what’s possible in sustainable building practices.
Real-Time Sustainability Monitoring Through Smart Analytics
Energy Consumption Optimization
Advanced AI systems are revolutionizing energy consumption monitoring and optimization in construction projects, delivering significant sustainability improvements and cost reductions. These intelligent systems analyze real-time data from IoT sensors and smart meters to create comprehensive energy usage profiles across construction sites.
Machine learning algorithms process this data to identify patterns and anomalies in energy consumption, enabling project managers to make informed decisions about equipment operation and site activities. For instance, AI-powered systems can detect inefficient equipment operation, unnecessary idle time, and peak usage periods, automatically adjusting power distribution to optimize consumption.
Recent implementations have shown remarkable results, with some construction sites reporting energy savings of up to 25% through AI-driven optimization. These systems excel at predicting energy demands based on project phases, weather conditions, and operational schedules, allowing for proactive resource allocation and reduced waste.
Key benefits include:
– Real-time monitoring and automated alerts for excessive energy use
– Predictive maintenance scheduling to ensure equipment efficiency
– Smart scheduling of high-energy activities during off-peak hours
– Automated adjustment of HVAC and lighting systems based on occupancy and weather
– Detailed reporting for compliance and sustainability certification requirements
Construction firms implementing these solutions have noted significant improvements in their environmental impact metrics while maintaining or improving project timelines. The integration of AI-driven energy optimization represents a crucial step toward achieving sustainable construction practices and meeting increasingly stringent environmental regulations.
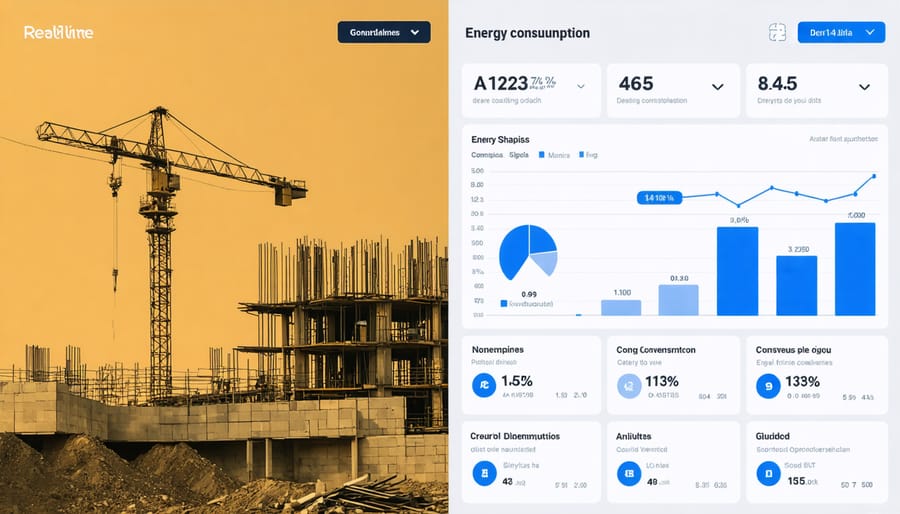
Waste Reduction Analytics
Data science is revolutionizing waste management in construction by enabling precise prediction and optimization of material usage. Through advanced predictive modeling and machine learning algorithms, construction companies can now forecast waste generation with unprecedented accuracy, supporting zero waste initiatives across projects of all scales.
By analyzing historical project data, material specifications, and construction methodologies, predictive models can identify patterns in waste generation and recommend optimal material ordering quantities. These analytics systems typically achieve 15-25% reduction in material waste, resulting in significant cost savings and environmental benefits.
Key applications include:
– Material requirement forecasting based on BIM data and project specifications
– Real-time waste tracking and optimization
– Automated inventory management systems
– Predictive maintenance to reduce equipment-related waste
Recent case studies demonstrate the effectiveness of these approaches. For instance, a major commercial development project in Singapore implemented waste reduction analytics and achieved a 30% decrease in concrete waste and a 22% reduction in overall material surplus. The system analyzed factors such as weather patterns, delivery schedules, and crew productivity to optimize material orders and usage timing.
Modern waste reduction analytics also incorporate IoT sensors and real-time monitoring to provide immediate feedback on material consumption patterns, enabling project managers to make data-driven decisions that support both sustainability goals and bottom-line objectives.
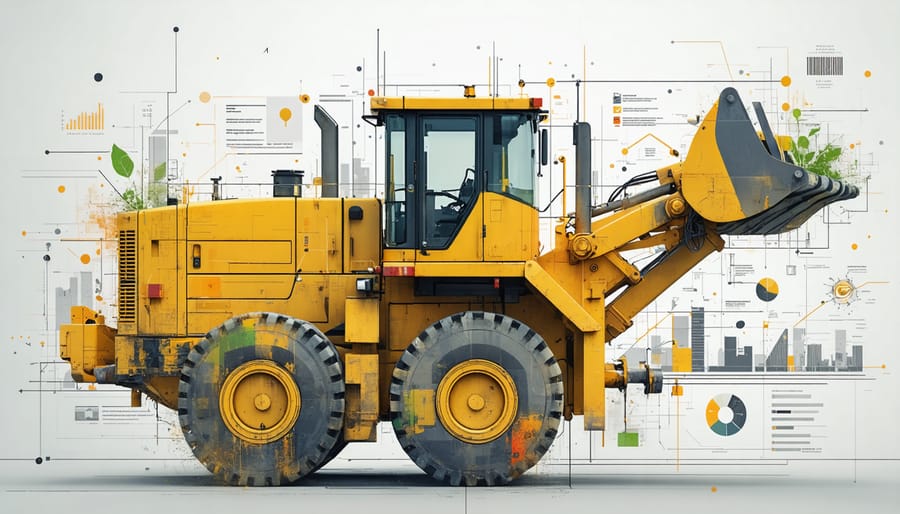
Predictive Maintenance for Sustainable Operations
Machine Learning in Equipment Lifecycle Management
Machine learning algorithms are revolutionizing equipment lifecycle management in construction, offering unprecedented opportunities for sustainable operations. By implementing smart digital tools, companies can now predict equipment failures before they occur, optimizing maintenance schedules and significantly reducing both downtime and unnecessary replacements.
These predictive maintenance systems analyze real-time sensor data, historical performance records, and environmental conditions to create accurate maintenance forecasts. For instance, ML algorithms can detect subtle changes in equipment vibration patterns, power consumption, or temperature variations that might indicate impending failures. This proactive approach extends equipment lifespan by 20-40% on average, while reducing energy consumption and waste generation.
The implementation of ML-driven maintenance strategies has shown remarkable results across various construction projects. Companies report up to 30% reduction in maintenance costs and a 25% decrease in equipment-related carbon emissions. These systems also help optimize equipment utilization rates, ensuring that machinery operates at peak efficiency levels while minimizing environmental impact.
By integrating these predictive maintenance capabilities with broader sustainability initiatives, construction firms can significantly improve their environmental performance while maintaining operational excellence.
Carbon Footprint Reduction Through Smart Maintenance
Smart maintenance powered by data science and AI is revolutionizing how construction companies approach equipment upkeep while significantly reducing embodied carbon in construction operations. Predictive maintenance algorithms analyze sensor data to optimize equipment performance, reducing unnecessary repairs and replacement cycles that contribute to carbon emissions.
Recent studies indicate that AI-driven maintenance can reduce equipment-related carbon emissions by up to 25% through improved efficiency and extended machinery lifespan. By preventing unexpected breakdowns and optimizing maintenance schedules, construction companies minimize the environmental impact of emergency repairs and parts transportation.
Machine learning models analyze historical performance data, environmental conditions, and usage patterns to predict potential failures before they occur. This proactive approach not only reduces downtime but also ensures equipment operates at peak efficiency, consuming less energy and producing fewer emissions.
Real-time monitoring systems track fuel consumption, emissions, and performance metrics, enabling immediate interventions when equipment operates outside optimal parameters. Construction firms implementing these systems report an average 15-20% reduction in fuel consumption and associated carbon emissions across their equipment fleet.
The integration of IoT sensors and data analytics platforms also enables better resource allocation, reducing unnecessary equipment deployment and associated environmental impacts.
Material Usage Optimization
Smart Material Selection Systems
Artificial Intelligence and machine learning algorithms are revolutionizing how construction professionals select sustainable building materials for their projects. These smart material selection systems analyze vast databases of material properties, environmental impact assessments, and life-cycle data to recommend optimal choices for specific construction requirements.
Advanced algorithms consider multiple variables simultaneously, including carbon footprint, durability, cost-effectiveness, local availability, and thermal performance. For instance, leading construction firms are implementing AI-powered platforms that can evaluate thousands of material combinations within seconds, providing data-driven recommendations that balance sustainability goals with project constraints.
These systems also incorporate real-time market data and supply chain information, enabling project managers to make informed decisions about material sourcing. The technology considers factors such as transportation distances, manufacturing processes, and end-of-life recyclability to calculate the true environmental impact of each material choice.
Recent implementations have shown remarkable results, with some projects achieving up to 30% reduction in embodied carbon through AI-optimized material selection. The systems continue to learn and improve through machine learning, adapting to new environmental standards and incorporating feedback from completed projects to refine their recommendation algorithms.
For project teams, these tools provide clear, quantifiable metrics for material sustainability, supporting evidence-based decision-making and helping meet increasingly stringent environmental regulations.
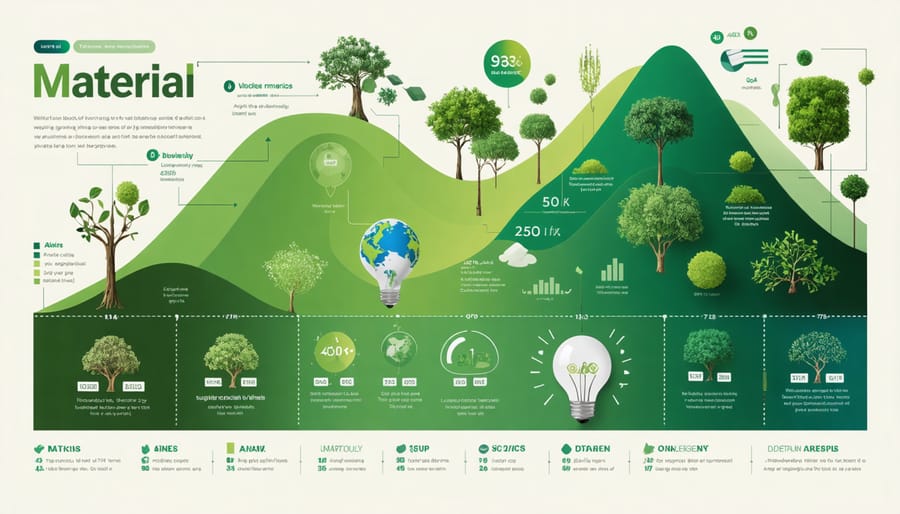
Waste Prevention Through Data Analysis
Predictive analytics is revolutionizing waste management in construction by enabling precise material ordering and usage patterns. Through sophisticated data analysis of historical project information, weather patterns, and supply chain metrics, construction firms can now forecast material requirements with unprecedented accuracy.
A recent industry study showed that data-driven material ordering reduced waste by up to 27% on large-scale projects. This approach combines multiple data streams, including BIM models, previous project statistics, and real-time site conditions, to create dynamic ordering schedules that minimize excess inventory while preventing shortages.
Key components of data-driven waste prevention include:
– Automated inventory tracking systems that monitor material usage in real-time
– Machine learning algorithms that predict material consumption rates based on project phase and type
– Integration with supplier databases to optimize delivery timing and quantities
– Weather pattern analysis to adjust material storage and usage schedules
Construction firms implementing these systems report significant benefits beyond waste reduction, including improved cash flow management and reduced storage costs. For example, Turner Construction’s implementation of predictive analytics resulted in a 15% reduction in material overordering and a 20% decrease in on-site storage requirements.
The system’s success relies on continuous data collection and analysis, allowing for increasingly accurate predictions as more project data becomes available. This iterative improvement process ensures that waste prevention strategies become more refined and effective over time.
Case Study: AI Analytics in Action
The Shanghai Sustainable Development Center project stands as a compelling example of AI-driven analytics transforming construction sustainability practices. In 2021, the development team partnered with leading AI specialists to implement a comprehensive sustainability monitoring system across the 250,000-square-meter mixed-use development.
The project utilized an integrated network of IoT sensors and AI algorithms to optimize energy consumption, reduce waste, and enhance building performance during both construction and operational phases. The system processed over 500,000 data points daily, analyzing everything from material usage patterns to energy consumption trends.
Key achievements of the AI implementation included:
• 27% reduction in construction waste through predictive material ordering
• 32% decrease in energy consumption during construction
• 45% improvement in construction schedule efficiency
• 18% reduction in carbon emissions compared to traditional methods
The AI system employed machine learning algorithms to analyze historical project data, weather patterns, and real-time construction progress. This enabled project managers to make data-driven decisions about resource allocation and scheduling. For instance, the system predicted optimal concrete pouring times based on weather forecasts and curing conditions, reducing material waste and improving quality outcomes.
The project’s success hinged on three critical components:
1. Real-time monitoring through a network of smart sensors
2. Advanced data analytics platform processing multiple data streams
3. AI-powered decision support system providing actionable insights
The construction team particularly benefited from the system’s ability to optimize material delivery schedules. By analyzing historical usage patterns and current progress rates, the AI predicted material requirements with 94% accuracy, significantly reducing on-site storage needs and associated waste.
Environmental impact monitoring was another crucial aspect. The AI system tracked carbon emissions throughout the construction process, enabling real-time adjustments to maintain sustainability targets. When emissions exceeded predetermined thresholds, the system automatically suggested alternative construction methods or materials.
The project team faced initial challenges in training staff to utilize the AI system effectively. However, investment in comprehensive training programs and user-friendly interfaces ensured successful adoption across all project levels. The resulting data-driven decision-making culture transformed how the team approached sustainable construction practices.
This case study demonstrates how AI analytics can deliver tangible sustainability improvements in large-scale construction projects. The success has led to the system’s implementation across multiple projects in the region, establishing a new benchmark for sustainable construction practices.
As the construction industry continues to evolve, the integration of AI-driven sustainability analytics presents both opportunities and imperatives for forward-thinking organizations. The convergence of data science and sustainability isn’t just a trend—it’s becoming a fundamental requirement for competitive advantage and environmental responsibility in construction.
To successfully implement AI-driven sustainability analytics, construction companies should begin by establishing a clear data strategy and infrastructure. This includes investing in IoT sensors, building management systems, and robust data collection protocols. Companies should also prioritize training their workforce in data literacy and creating dedicated teams that bridge the gap between construction expertise and data science capabilities.
The roadmap to implementation should follow a phased approach: starting with pilot projects in specific areas such as energy optimization or waste reduction, then scaling successful initiatives across operations. It’s crucial to establish clear metrics for success and maintain transparent reporting mechanisms to track progress and ROI.
Looking ahead, we can expect to see more sophisticated applications of machine learning in sustainable construction, including predictive maintenance systems that optimize building performance and automated decision-making tools for material selection and resource allocation. Construction companies that embrace these technologies now will be better positioned to meet increasingly stringent environmental regulations and growing client demands for sustainable building solutions.
Success in this transformation requires commitment from leadership, investment in technology infrastructure, and a culture that values data-driven decision-making. By taking these steps today, construction companies can build a more sustainable future while maintaining competitive advantage in an evolving market.