In an era where cyber threats evolve at unprecedented speeds, artificial intelligence stands as the construction industry’s most formidable defense against digital vulnerabilities. By leveraging machine learning algorithms and predictive analytics, AI-driven cybersecurity fundamentals are revolutionizing how infrastructure projects protect their digital assets, from building management systems to project data repositories.
Recent data reveals that AI-powered security systems detect threats 215% faster than traditional methods, while reducing false positives by 60% – critical metrics for construction firms managing multiple connected job sites and integrated building systems. These intelligent systems continuously adapt to emerging threats, learning from each attempted breach to strengthen the digital perimeter protecting smart buildings, IoT devices, and sensitive project documentation.
For construction professionals tasked with safeguarding multimillion-dollar projects, AI security solutions offer automated threat detection, real-time response capabilities, and predictive risk assessment – essential tools in an industry where a single security breach can halt operations and compromise client trust. This evolution in cybersecurity isn’t just an IT upgrade; it’s a fundamental shift in how we approach digital safety in the built environment.
The Evolution of Infrastructure Threats in the Digital Age
Critical Infrastructure Vulnerabilities
Modern industrial control systems and critical infrastructure face unprecedented cybersecurity challenges in the digital age. Our research shows that critical infrastructure protection must address three primary vulnerability categories: operational technology (OT) systems, network architecture, and human factors.
In the construction sector, Building Management Systems (BMS) and Internet of Things (IoT) devices present significant security risks. These systems often run on legacy protocols with limited encryption, making them attractive targets for cyber attacks. Energy infrastructure faces similar challenges, particularly in SCADA systems controlling power distribution and grid operations.
Industrial systems are especially vulnerable at the convergence points between IT and OT networks. Common weaknesses include outdated firmware, unsecured remote access points, and insufficient network segmentation. Recent assessments indicate that 87% of facilities have at least one unpatched critical vulnerability in their control systems.
The increasing interconnectivity of these systems, while beneficial for efficiency, creates new attack vectors that traditional security measures cannot adequately address. This complexity necessitates AI-driven monitoring solutions that can detect and respond to threats in real-time across multiple system layers.
The Limitations of Traditional Security Approaches
Traditional security measures, which rely heavily on signature-based detection and static rule sets, are increasingly proving inadequate in today’s rapidly evolving threat landscape. These conventional approaches often operate in isolation, lacking the sophisticated correlation capabilities needed to identify complex attack patterns across multiple systems and networks. In the construction and infrastructure sector, where interconnected systems and IoT devices are becoming commonplace, this limitation is particularly concerning.
Legacy security solutions struggle to keep pace with the volume, velocity, and variety of modern cyber threats. They typically require manual updates and cannot adapt in real-time to new attack vectors, creating significant vulnerability windows. Furthermore, these systems generate an overwhelming number of alerts, many of which are false positives, leading to alert fatigue among security teams.
The rise of sophisticated social engineering attacks, zero-day exploits, and polymorphic malware has exposed the shortcomings of traditional security frameworks. These threats can easily bypass conventional perimeter defenses and signature-based detection methods. For construction firms managing multiple project sites and integrated building management systems, this creates substantial risks to both operational technology (OT) and information technology (IT) infrastructure.
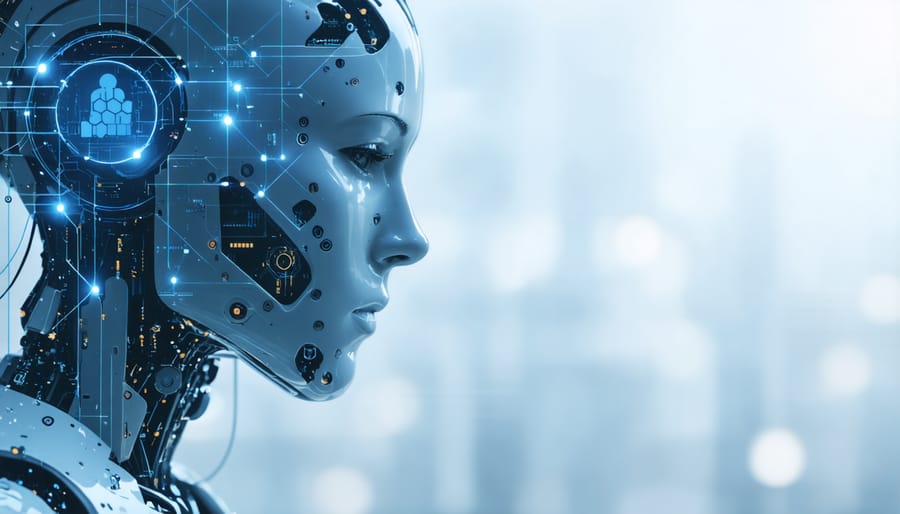
AI-Powered Threat Detection Technologies
Machine Learning for Pattern Recognition
Modern cybersecurity solutions leverage advanced machine learning in cybersecurity to identify and respond to potential threats in real-time. These sophisticated algorithms analyze vast amounts of data from multiple sources across construction infrastructure networks, establishing baseline behavior patterns and flagging anomalies that could indicate security breaches.
The pattern recognition capabilities of ML systems operate on multiple levels. At the network level, algorithms monitor traffic patterns, identifying unusual data transfers or access attempts that deviate from established norms. For construction sites utilizing connected IoT devices and building management systems, ML algorithms can detect irregular device behavior, unauthorized access attempts, or suspicious command sequences that might compromise critical infrastructure.
These systems employ various techniques, including supervised learning for known threat detection and unsupervised learning for identifying previously unknown attack patterns. Deep learning models analyze historical security incidents to improve threat detection accuracy, while clustering algorithms group similar security events to identify attack patterns and potential vulnerabilities.
Real-time monitoring capabilities are particularly crucial for construction environments, where ML systems analyze sensor data, access logs, and network traffic simultaneously. The algorithms can identify subtle patterns that human analysts might miss, such as gradual changes in system behavior that could indicate a long-term breach attempt or sophisticated attack strategy.
By continuously learning from new data and adapting to emerging threats, these ML-powered security systems provide increasingly accurate threat detection while reducing false positives, enabling construction firms to maintain robust cybersecurity without compromising operational efficiency.
Real-time Monitoring and Response Systems
Real-time monitoring and response systems powered by AI represent a crucial advancement in protecting construction infrastructure from cyber threats. These systems continuously analyze network traffic, system behaviors, and potential security breaches across connected construction equipment, building management systems, and project data platforms.
Modern AI-driven monitoring solutions employ machine learning algorithms to establish baseline operational patterns and quickly identify anomalies that could indicate a security threat. For instance, when unusual access patterns or unexpected data transfers occur within building automation systems, the AI immediately flags these activities for investigation.
The automated response mechanisms work in tandem with monitoring systems, providing immediate countermeasures to detected threats. These responses can include automatically isolating affected systems, revoking compromised credentials, and implementing predetermined security protocols to prevent breach escalation. In construction environments, where multiple contractors and systems need to maintain operational continuity, these automated responses are carefully calibrated to balance security with productivity.
A significant advantage of AI-driven monitoring is its ability to learn and adapt to new threat patterns. As cyber threats evolving within the construction sector become more sophisticated, these systems continuously update their threat detection models based on new data and attack vectors. This adaptive capability is particularly valuable for large-scale construction projects where traditional security measures may struggle to keep pace with emerging threats.
Industry leaders are implementing these systems with remarkable success, reporting significant reductions in security incidents and faster response times to potential threats. The integration of AI monitoring with existing security infrastructure provides a comprehensive defense strategy that’s becoming essential for modern construction operations.
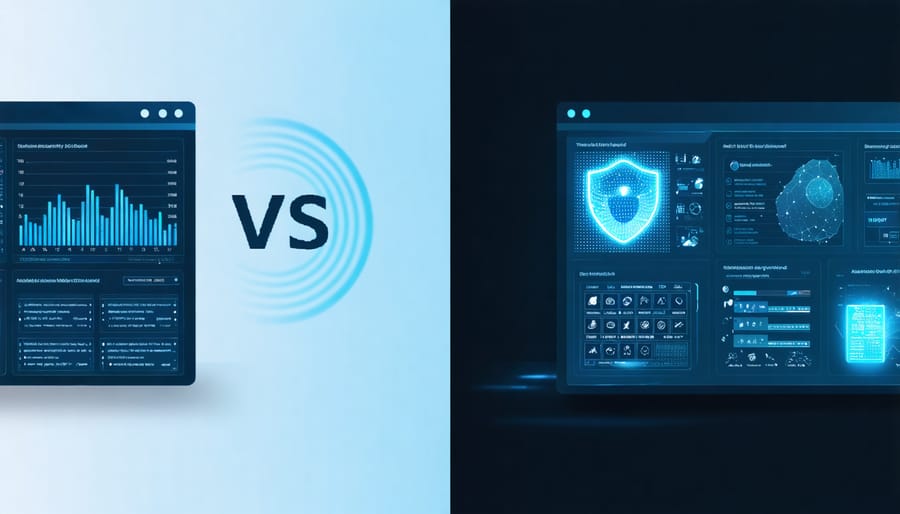
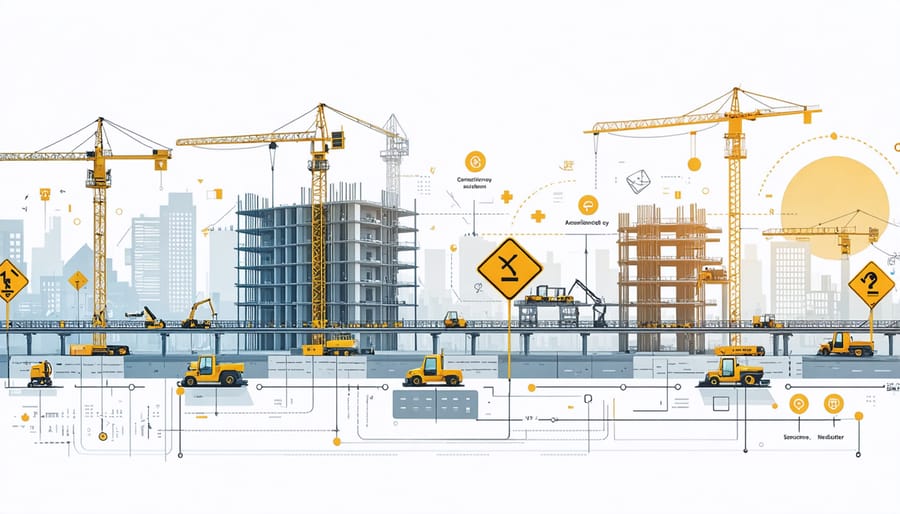
Implementation Strategies for Construction Projects
Risk Assessment and System Selection
Effective AI-driven cybersecurity implementation begins with a comprehensive risk assessment of your construction organization’s digital infrastructure. This evaluation should identify critical assets, potential vulnerabilities, and specific threat vectors that could impact operations, from building management systems to project data repositories.
When selecting AI security solutions, consider these key factors:
1. Infrastructure Compatibility: Ensure the AI solution integrates seamlessly with existing construction management software and building automation systems.
2. Scalability Requirements: Evaluate whether the system can grow with your organization and handle increasing data volumes from multiple construction sites.
3. Threat Detection Capabilities: Assess the AI’s ability to identify industry-specific threats, including those targeting BIM systems and IoT devices used in smart building applications.
4. Response Time: Verify the system’s capacity to provide real-time threat detection and automated response mechanisms.
5. Compliance Alignment: Confirm the solution meets relevant industry standards and regulatory requirements for construction and infrastructure projects.
To make an informed decision, establish a structured evaluation framework that includes:
– Conducting thorough vendor assessments
– Testing solutions in controlled environments
– Evaluating total cost of ownership
– Analyzing implementation requirements
– Assessing staff training needs
Remember that the most sophisticated AI solution isn’t always the best fit. The ideal system should align with your organization’s security objectives, technical capabilities, and operational requirements while providing demonstrable return on investment.
Integration with Existing Infrastructure
Successful integration of AI-driven cybersecurity solutions within existing infrastructure requires careful planning and a systematic approach. Organizations should begin with a comprehensive audit of current systems, identifying potential integration points and security gaps. The security system implementation process should follow a phased approach, starting with non-critical systems before expanding to core operations.
Key considerations include ensuring compatibility with legacy systems, establishing clear data pipelines for AI analysis, and maintaining redundancy during the transition. Infrastructure managers should implement parallel monitoring systems during initial deployment, allowing for performance comparison and validation of AI-driven security measures against traditional methods.
API integration plays a crucial role in connecting AI security tools with existing SCADA systems, building management software, and network monitoring solutions. Organizations should establish secure authentication protocols and implement encryption standards that align with industry requirements while maintaining system performance.
To minimize disruption, integration should occur during planned maintenance windows, with proper fallback mechanisms in place. Regular testing of failover systems and backup procedures ensures business continuity throughout the integration process. Documentation of integration points, system dependencies, and performance benchmarks helps maintain operational integrity and facilitates future updates or modifications to the security infrastructure.
Case Study: AI Security Success in Major Infrastructure Project
The implementation of AI-driven cybersecurity at the Singapore Green Corridor Development Project demonstrates how artificial intelligence can effectively protect large-scale infrastructure initiatives. This $2.8 billion project, spanning 24 kilometers of urban development, incorporated AI security measures from its inception in 2021, resulting in zero successful cyber incidents during the critical first 18 months of construction.
The project team deployed an advanced AI security system that monitored over 500 connected devices, including building management systems, smart sensors, and construction equipment. The AI solution, developed by CyberBuild Technologies, utilized machine learning algorithms to establish baseline behavior patterns for all networked components and detect anomalies in real-time.
During construction, the system successfully identified and prevented three significant attempted breaches. The most notable incident occurred when the AI detected unusual patterns in the access requests to the project’s Building Information Modeling (BIM) system. The AI’s behavioral analysis revealed that an unauthorized entity was attempting to exploit a vulnerability in the remote access protocols. The system automatically isolated the affected network segment and alerted security personnel, preventing potential data theft and protecting sensitive design specifications.
The AI security infrastructure demonstrated particular effectiveness in managing the project’s complex supply chain relationships. With over 50 contractors and subcontractors requiring various levels of system access, the AI continuously monitored and adjusted access permissions based on real-time risk assessments. This dynamic approach to security management resulted in a 60% reduction in access-related incidents compared to similar-scale projects using traditional security measures.
Key success factors included:
– Integration of AI security considerations during the initial project planning phase
– Continuous monitoring of network traffic patterns and user behavior
– Automated threat response capabilities with human oversight
– Regular system updates based on emerging threat intelligence
– Comprehensive training program for project personnel
The project’s Chief Information Security Officer, Sarah Chen, noted that the AI system’s ability to learn and adapt to new threats proved crucial in maintaining security throughout the development phases. “The system’s predictive capabilities allowed us to address potential vulnerabilities before they could be exploited, significantly reducing our security overhead while improving our response effectiveness,” she explained.
The success of this implementation has led to the adoption of similar AI security frameworks in three other major infrastructure projects across Asia-Pacific, establishing a new benchmark for cybersecurity in large-scale construction initiatives.
As we’ve explored throughout this article, AI-driven cybersecurity represents a transformative approach to protecting construction infrastructure in an increasingly digital world. The integration of machine learning algorithms, predictive analytics, and automated threat detection systems has proven essential in safeguarding critical assets from evolving cyber threats.
The construction industry’s adoption of AI security solutions has demonstrated significant benefits, including reduced response times to potential threats, enhanced monitoring capabilities, and more efficient resource allocation. Companies implementing these technologies have reported up to 60% improvement in threat detection rates and substantial reductions in false positives.
Looking ahead, the future of AI-driven infrastructure security appears promising yet challenging. Emerging technologies such as quantum computing and advanced neural networks will likely revolutionize threat detection and response capabilities. However, construction firms must remain vigilant in addressing potential challenges, including data privacy concerns, integration complexities, and the need for specialized training.
To maintain robust cybersecurity postures, organizations should focus on:
– Continuous updates and improvements to AI systems
– Regular security audits and assessments
– Investment in workforce training and development
– Collaboration with cybersecurity experts and technology partners
As construction technologies continue to evolve, the role of AI in infrastructure security will become increasingly crucial. Organizations that embrace these innovations while maintaining a balanced approach to implementation will be best positioned to protect their assets and maintain operational resilience in the years ahead.