In an era where AI is revolutionizing construction, predictive maintenance has emerged as a cornerstone of operational efficiency. Advanced machine learning algorithms now analyze real-time sensor data from construction equipment, predicting failures before they occur and reducing downtime by up to 50%. This technological transformation extends beyond mere maintenance—AI-powered systems optimize project schedules, enhance safety protocols, and deliver unprecedented accuracy in cost estimation.
For construction leaders navigating this digital evolution, the integration of AI represents both a significant opportunity and a strategic imperative. From autonomous equipment that operates with precision to intelligent project management platforms that process millions of data points instantaneously, artificial intelligence is redefining industry standards. Recent studies indicate that AI implementation in construction can increase productivity by 40% and reduce project costs by 20%, making it an essential consideration for forward-thinking organizations.
This comprehensive analysis explores how AI is reshaping predictive maintenance in construction, offering practical insights for implementation and examining real-world applications that demonstrate measurable returns on investment.
The Evolution from Reactive to Predictive Maintenance
Traditional Maintenance Challenges
Traditional maintenance approaches in the construction industry have long been plagued by significant inefficiencies and limitations. Reactive maintenance, which addresses issues only after equipment failure, leads to costly emergency repairs, extended downtime, and disrupted project schedules. This approach often results in 30-40% higher maintenance costs compared to planned maintenance strategies.
Preventive maintenance, while more structured, presents its own challenges. Based on fixed schedules rather than actual equipment condition, this approach frequently results in unnecessary maintenance interventions and parts replacement. Industry data suggests that up to 30% of preventive maintenance activities are performed prematurely, leading to wasted resources and reduced equipment lifespan.
Both approaches suffer from limited visibility into equipment health, making it difficult to optimize maintenance scheduling and resource allocation. The lack of real-time monitoring capabilities means that subtle signs of deterioration often go unnoticed until they escalate into major issues. Additionally, these traditional methods struggle with documentation accuracy and knowledge retention, as maintenance histories are often incomplete or poorly organized, hampering long-term equipment performance analysis and improvement strategies.
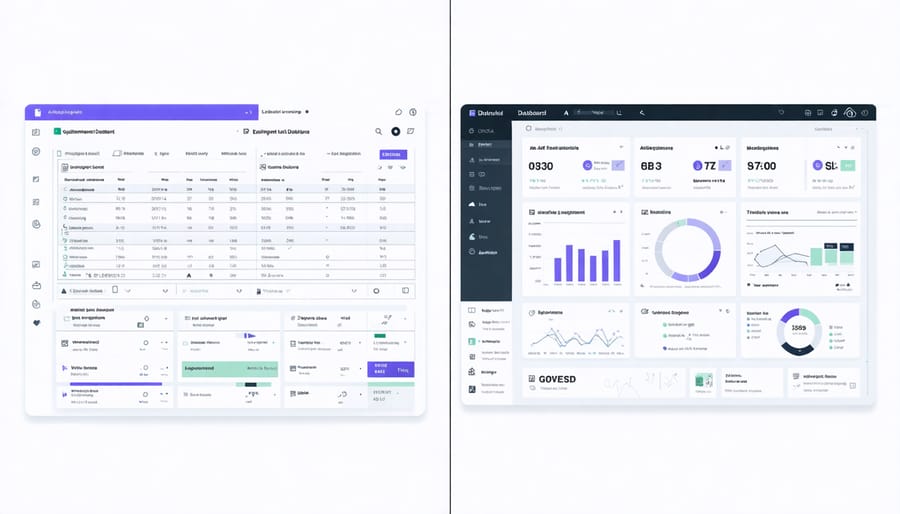
The AI Advantage
Artificial Intelligence is revolutionizing maintenance strategies in construction by shifting from reactive to predictive approaches. Through advanced algorithms and machine learning capabilities, AI systems continuously analyze data from sensors, equipment logs, and historical maintenance records to identify potential issues before they become critical failures.
This predictive capability enables construction companies to optimize maintenance schedules, reduce downtime, and extend equipment lifespan. AI systems can detect subtle changes in equipment performance patterns, from minor vibration anomalies to temperature fluctuations, providing early warning signs of potential failures.
The technology also excels at resource optimization, automatically generating maintenance schedules that balance equipment availability with operational demands. By analyzing patterns across multiple projects and equipment types, AI can recommend the most cost-effective maintenance interventions and predict parts replacement needs with remarkable accuracy.
Construction firms implementing AI-driven maintenance strategies report up to 30% reduction in maintenance costs and a 25% decrease in unexpected equipment failures. These improvements translate directly to enhanced project timelines, reduced operational risks, and improved safety conditions on construction sites.
Core Components of AI Predictive Maintenance
Sensor Integration and IoT
The integration of IoT sensors and data collection systems has revolutionized how construction projects are monitored and managed. Advanced sensor networks now provide real-time data on structural integrity, environmental conditions, equipment performance, and worker safety across construction sites. These smart sensors continuously monitor critical parameters such as temperature, humidity, vibration, strain, and displacement, creating a comprehensive digital overview of project conditions.
Modern construction sites typically employ a multi-layered sensor infrastructure, including embedded structural sensors, environmental monitoring devices, and wearable technology for workers. This data collection network feeds into centralized platforms where AI algorithms analyze the information to identify patterns, predict potential issues, and optimize operations.
The implementation of IoT sensors has proven particularly valuable in large-scale projects, where they enable remote monitoring of multiple sites simultaneously. For instance, sensors can detect minute changes in concrete curing conditions, monitor equipment utilization rates, and track material movement across the site. This granular level of monitoring not only enhances safety and quality control but also provides valuable insights for predictive maintenance and resource allocation, ultimately reducing downtime and improving project efficiency.
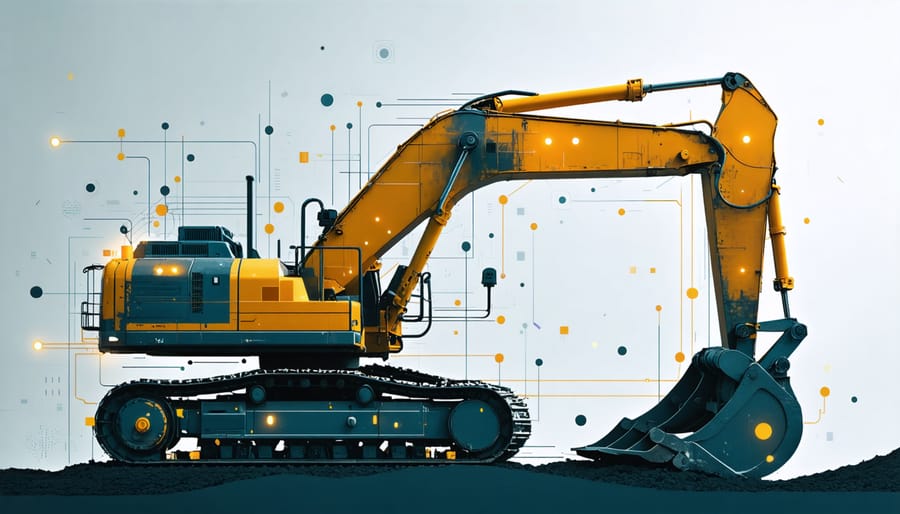
Machine Learning Algorithms
Several machine learning algorithms are transforming how construction projects are managed and executed. Supervised learning models, particularly Random Forests and Support Vector Machines (SVMs), excel at predicting project timelines and cost overruns by analyzing historical project data. These algorithms can process multiple variables simultaneously, from weather patterns to material costs, providing accurate forecasts for project planning.
Deep Learning neural networks have proven particularly effective in image recognition applications, enabling automated progress monitoring and safety compliance checking on construction sites. Convolutional Neural Networks (CNs) can analyze site photos and video feeds to detect safety violations or track construction progress in real-time.
Reinforcement learning algorithms are increasingly being applied to optimize construction schedules and resource allocation. These systems learn from past project outcomes to suggest optimal sequences for construction tasks, while data analysis in construction continues to evolve with more sophisticated predictive capabilities.
Natural Language Processing (NLP) algorithms help in analyzing construction documents, contracts, and specifications, automating the extraction of critical information and identifying potential conflicts or risks before they materialize.
Real-time Monitoring Systems
Real-time monitoring systems powered by AI have revolutionized how construction companies track and maintain their equipment fleet. These sophisticated systems utilize IoT sensors and advanced analytics to continuously collect and process data from various equipment components, enabling unprecedented visibility into machine health and performance. Among the latest construction site monitoring innovations, AI-driven systems can detect subtle variations in equipment performance parameters, including vibration patterns, temperature fluctuations, and operational metrics.
The technology employs machine learning algorithms to establish baseline performance patterns and identify deviations that might indicate potential issues. This proactive approach allows maintenance teams to address problems before they escalate into costly breakdowns. Real-time alerts and detailed performance analytics are delivered through user-friendly dashboards, enabling project managers and maintenance personnel to make data-driven decisions quickly.
These systems also integrate with existing maintenance management platforms, creating a comprehensive ecosystem for equipment oversight. The result is optimized maintenance scheduling, reduced downtime, and significant cost savings through the prevention of major equipment failures.
Real-World Implementation Success Stories
Large-Scale Infrastructure Project
The Dubai Creek Tower project serves as a compelling example of AI integration in large-scale construction. This ambitious development leveraged artificial intelligence across multiple phases, resulting in a 23% reduction in overall construction time and a 15% decrease in material waste.
The project team implemented AI-powered Building Information Modeling (BIM) systems to create detailed 3D visualizations and simulate construction sequences. Machine learning algorithms analyzed thousands of variables, including weather patterns, material properties, and structural loads, to optimize the construction schedule and resource allocation.
Autonomous drones equipped with LiDAR sensors conducted daily site surveys, generating precise point clouds that were compared against the digital twin model. This real-time monitoring enabled early detection of potential issues and automated progress tracking, with an accuracy rate of 98.5%.
The project’s success hinged on its innovative use of AI for logistics management. Smart sensors and IoT devices monitored material movement and equipment utilization, while predictive analytics optimized delivery schedules and crane operations. This resulted in a 30% improvement in equipment efficiency and a significant reduction in idle time.
Risk management was enhanced through AI-driven predictive modeling, which identified potential safety hazards and structural challenges before they materialized. The system processed data from multiple sources, including weather forecasts, soil conditions, and structural stress measurements, to provide actionable insights for the project team.
ROI Analysis
The implementation of AI in construction projects has demonstrated significant returns on investment across multiple dimensions. According to recent industry studies, construction companies utilizing AI-powered solutions report an average cost reduction of 15-20% in project expenses and a 20-25% improvement in project delivery timelines.
One notable area of financial benefit is in predictive maintenance, where AI systems have shown to reduce equipment downtime by up to 35% and maintenance costs by 25%. These savings are particularly impactful for large-scale projects where equipment failures can cost thousands of dollars per hour in delays.
Resource allocation optimization through AI has yielded impressive results, with companies reporting labor cost savings of 10-15% through better workforce scheduling and material waste reduction of up to 25%. Advanced AI algorithms help predict material requirements more accurately, minimizing overordering and storage costs.
Risk mitigation represents another significant area of cost savings. AI-powered safety systems have reduced accident-related costs by up to 30% in some cases, while predictive analytics have helped companies avoid costly delays by identifying potential issues before they escalate.
The initial investment in AI technology typically ranges from $50,000 to $500,000, depending on project scale and complexity. However, most companies achieve positive ROI within 12-18 months of implementation, with some reporting payback periods as short as 6 months for specific applications like automated quality control systems.
Implementation Strategies and Best Practices
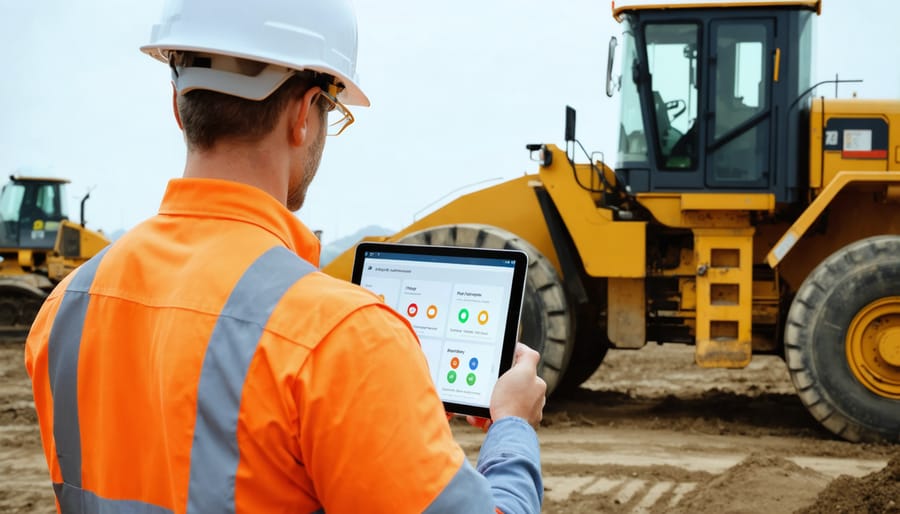
Getting Started
Implementing AI predictive maintenance in construction begins with a systematic approach to data collection and system evaluation. Start by conducting a comprehensive audit of your existing maintenance processes and equipment fleet. Identify critical assets that would benefit most from predictive monitoring, such as heavy machinery, HVAC systems, and structural components.
Next, establish a robust data infrastructure by installing appropriate sensors and monitoring devices on selected equipment. These sensors should track key performance indicators like vibration patterns, temperature fluctuations, and operational hours. Ensure your team has the necessary connectivity infrastructure to support real-time data transmission.
Partner with an AI solutions provider experienced in construction applications to develop or customize predictive algorithms. Begin with a pilot program focused on a specific asset class or project site to minimize risk and validate the system’s effectiveness. During this phase, train your maintenance staff on the new technology and establish clear protocols for responding to AI-generated alerts.
Document baseline performance metrics before implementation to measure the system’s impact accurately. Create a feedback loop between maintenance teams and AI systems to continuously improve prediction accuracy and maintenance recommendations.
Common Challenges
While AI offers tremendous potential in construction, several challenges must be addressed for successful implementation. Data quality and consistency remain primary concerns, as AI systems require large volumes of accurate, standardized data to function effectively. Many construction firms struggle with fragmented data collection processes and incompatible legacy systems.
Integration costs pose another significant hurdle, particularly for smaller companies. The initial investment in AI technology, infrastructure upgrades, and staff training can be substantial. Additionally, resistance to change among workers and stakeholders can impede adoption, often stemming from concerns about job security and unfamiliarity with new technologies.
Technical expertise gaps present ongoing challenges, as construction companies need specialists who understand both AI technology and construction processes. Finding or developing this talent can be time-consuming and expensive.
To overcome these obstacles, organizations should focus on phased implementation approaches, comprehensive training programs, and clear communication about AI’s role in augmenting rather than replacing human workers. Establishing partnerships with technology providers and investing in data management infrastructure can help address technical challenges while maintaining cost-effectiveness.
Future Integration Considerations
As the construction industry continues to embrace digital construction technology, future integration of AI systems will require careful planning and strategic foresight. Organizations must consider developing scalable infrastructure that can accommodate emerging technologies and increasing data volumes. This includes establishing robust cloud computing capabilities, implementing standardized data collection protocols, and ensuring system interoperability across different platforms and tools.
Key considerations should focus on training programs for workforce adaptation, regular technology assessments to identify upgrade opportunities, and maintaining flexibility in system architecture to integrate new AI capabilities as they emerge. Construction firms should also plan for increased cybersecurity measures, as AI systems become more sophisticated and interconnected.
Looking ahead, successful AI integration will depend on creating clear governance frameworks, establishing data sharing protocols between stakeholders, and developing standardized metrics for measuring AI performance and ROI. Companies should also consider forming strategic partnerships with technology providers to ensure long-term support and continuous innovation in their AI implementations.
As we’ve explored throughout this article, AI-powered predictive maintenance represents a transformative opportunity for the construction industry. By implementing these advanced systems, construction companies can achieve substantial reductions in equipment downtime, maintenance costs, and unexpected failures. The data-driven insights provided by AI algorithms enable teams to transition from reactive to proactive maintenance strategies, ultimately extending asset lifespans and optimizing resource allocation.
The benefits are clear: increased operational efficiency, enhanced safety protocols, and significant cost savings. Companies that have adopted AI predictive maintenance report up to 30% reduction in maintenance costs and 70% decrease in breakdowns. These improvements directly impact project timelines and bottom-line results, making AI adoption a strategic imperative rather than just a technological upgrade.
Looking ahead, the construction industry stands at a crucial junction. Early adopters of AI predictive maintenance are already gaining competitive advantages through improved equipment reliability and operational efficiency. For those yet to implement these solutions, the time to act is now. The technology has matured, implementation costs have decreased, and the return on investment is well-documented.
We encourage construction professionals to begin their AI journey by starting with pilot programs on critical equipment, gradually expanding their implementation based on results and lessons learned. The future of construction maintenance is predictive, data-driven, and intelligent – embrace it today to secure your company’s position in tomorrow’s construction landscape.