Data analysis is transforming the construction industry, empowering companies to make smarter decisions, reduce risks, and optimize project outcomes. By leveraging the power of construction data, firms can gain unprecedented insights into every aspect of their operations, from planning and design to execution and maintenance. This article explores how advanced analytics tools and techniques are being applied across the construction value chain to drive efficiency, productivity, and profitability. Through real-world case studies and expert insights, we’ll showcase the transformative potential of data-driven approaches and provide practical guidance for industry professionals looking to harness the power of analytics in their projects. Whether you’re a seasoned executive or an aspiring innovator, this comprehensive guide will equip you with the knowledge and strategies needed to thrive in the era of construction data analytics.
Improved Project Planning and Scheduling
Data analytics is revolutionizing project planning and scheduling in the construction industry. By leveraging vast amounts of historical data, construction firms can develop more accurate project timelines, optimize resource allocation, and streamline logistics. Predictive analytics tools analyze past project data to identify patterns and trends, enabling project managers to anticipate potential delays and plan accordingly. This data-driven approach helps minimize schedule overruns and ensures timely project completion.
Moreover, data analytics facilitates efficient resource allocation by providing insights into labor, equipment, and material requirements. By analyzing real-time data from sensors and IoT devices, project managers can track resource utilization and make informed decisions to optimize productivity. For instance, Komatsu, a leading construction equipment manufacturer, has developed a fleet monitoring system that collects data from sensors on their machines. This data is analyzed to predict maintenance needs, reduce downtime, and optimize equipment deployment across construction sites.
In terms of logistics, data analytics helps streamline supply chain management and inventory control. By analyzing supplier performance data, construction firms can identify reliable vendors, negotiate better contracts, and ensure timely delivery of materials. Predictive analytics can also forecast demand for materials, allowing companies to optimize inventory levels and minimize waste. For example, Skanska, a global construction company, has implemented a cloud-based platform that integrates data from various sources to provide real-time visibility into their supply chain. This has enabled them to reduce inventory costs and improve material availability on their projects.
Furthermore, data analytics enables construction firms to optimize site logistics by analyzing traffic patterns, weather conditions, and other external factors. By integrating this data with project schedules, managers can make informed decisions to minimize disruptions and ensure smooth operations on site. Advanced analytics tools can also simulate various scenarios to identify potential bottlenecks and develop contingency plans.
As the construction industry continues to embrace digital transformation, data analytics will play an increasingly crucial role in project planning and scheduling. By leveraging the power of data, construction firms can optimize their operations, reduce delays, and deliver projects more efficiently.
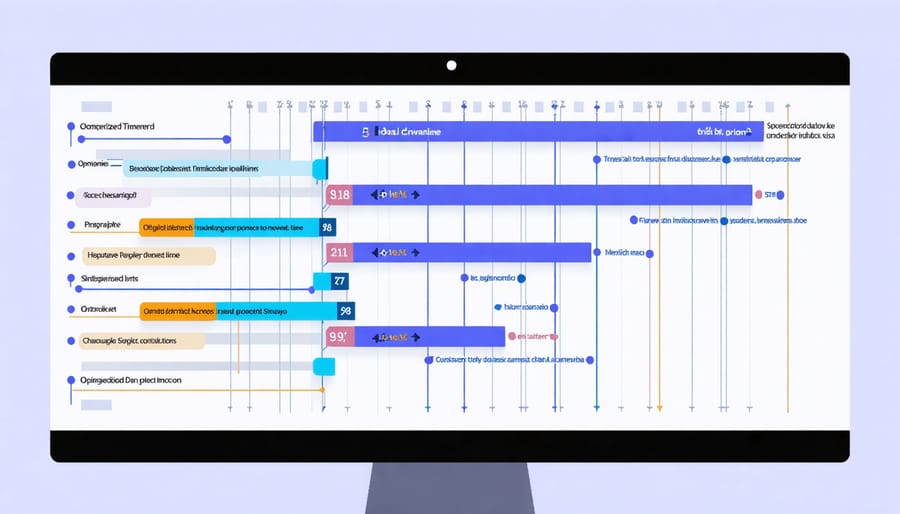
Enhanced Risk Management
In the construction industry, risk management is a critical component of successful project delivery. However, traditional risk management approaches often rely on historical data and subjective assessments, which can be inadequate in today’s complex construction landscape. This is where predictive analytics comes into play. By leveraging advanced data analysis techniques, construction professionals can identify and mitigate potential risks before they materialize, thereby minimizing their impact on project timelines, budgets, and quality.
Predictive analytics utilizes sophisticated algorithms and machine learning models to analyze vast amounts of data from various sources, such as project schedules, financial records, weather patterns, and supplier performance metrics. By identifying patterns and correlations within this data, the models can forecast potential risks, such as delays, cost overruns, safety incidents, and quality issues. Armed with these insights, project managers can proactively implement risk mitigation strategies, such as adjusting resource allocation, renegotiating contracts, or modifying construction methods.
Real-world case studies demonstrate the effectiveness of predictive analytics in risk management. For example, a large construction firm used predictive modeling to analyze data from over 500 projects and identified a high likelihood of delays in a particular project due to supplier performance issues. By proactively addressing these issues with the supplier and developing contingency plans, the firm was able to avoid a potential 3-month delay and $2 million in additional costs.
As the construction industry continues to embrace digital transformation, the adoption of predictive analytics for risk management is expected to grow. However, successfully implementing these techniques requires a strong data governance framework, skilled data analysts, and buy-in from stakeholders across the organization. By overcoming these challenges, construction companies can harness the power of predictive analytics to enhance risk management and deliver projects more efficiently and effectively.
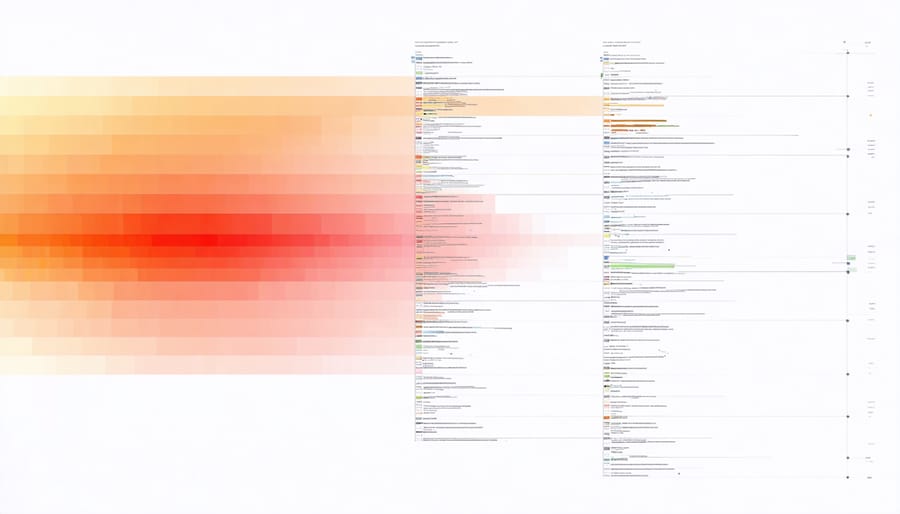
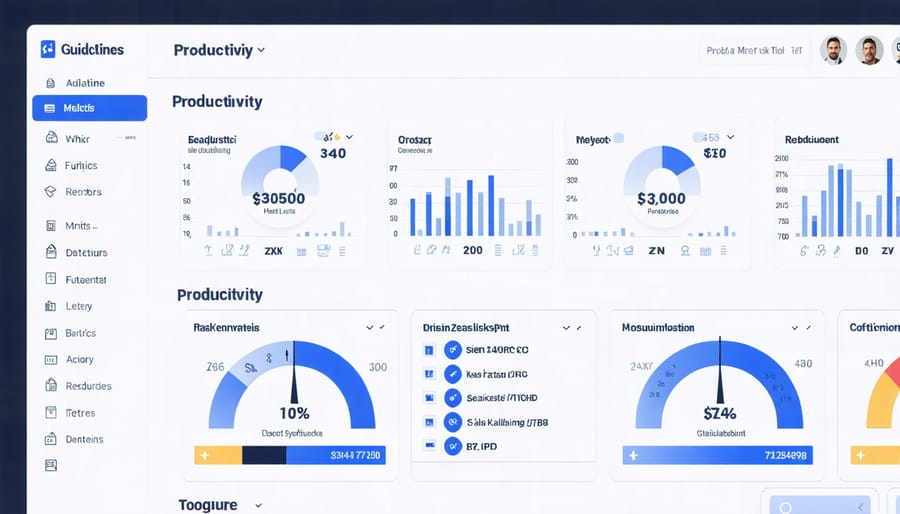
Boosting Productivity and Efficiency
Equipment Optimization
Data analysis is revolutionizing equipment management in construction. By leveraging sensor data from IoT devices, companies can optimize equipment usage, predict maintenance needs, and reduce fuel consumption. Sensors track metrics like operating hours, idle time, and performance, enabling data-driven decisions. Predictive maintenance algorithms analyze this data to identify potential issues before they cause downtime, saving costs and improving reliability. Fuel consumption data helps optimize routes and identify inefficient practices, leading to significant cost savings and reduced environmental impact. Case studies demonstrate the tangible benefits of these approaches. For example, a leading construction firm saved 15% on equipment costs and increased uptime by 10% after implementing a data-driven optimization strategy. As more companies adopt these technologies, equipment optimization will become a key competitive advantage in the industry.
Labor Productivity Tracking
Analyzing worker performance data is crucial for optimizing labor productivity in construction projects. By tracking metrics such as task completion times, idle time, and rework, project managers can gain valuable insights into crew efficiency. This data enables the identification of skill gaps and training needs, allowing for targeted interventions to improve worker capabilities. Moreover, data-driven crew assignments ensure that the right workers are allocated to the tasks they excel at, maximizing productivity. Predictive analytics can even forecast potential productivity bottlenecks, enabling proactive mitigation strategies. Real-time monitoring using IoT devices and wearables further enhances data collection, providing a granular view of worker performance. By leveraging these insights, construction firms can optimize labor utilization, reduce costs, and improve project outcomes. As the industry adopts more advanced analytics tools, labor productivity tracking will become an increasingly critical aspect of data-driven construction management.
Smarter Building Design
Data analysis is revolutionizing building design by integrating with Building Information Modeling (BIM) and generative design tools. By leveraging vast amounts of data from past projects, environmental factors, and building performance simulations, architects and engineers can optimize designs for energy efficiency, occupant comfort, and sustainability.
One powerful application is the use of digital twins, which are virtual replicas of physical buildings that can be analyzed and tested under various conditions. By feeding real-time data into these models, designers can predict how a building will perform and make informed decisions to improve its efficiency and functionality.
Generative design algorithms, powered by machine learning, can explore thousands of design variations based on predefined parameters and constraints. These algorithms consider factors such as building orientation, materials, and space utilization to generate optimal designs that meet specific performance criteria. This data-driven approach leads to more innovative and efficient building solutions that may not have been considered through traditional design methods.
By integrating data analysis into the design process, architects and engineers can create buildings that are not only aesthetically pleasing but also highly functional and sustainable. This smarter approach to building design ultimately results in reduced energy consumption, lower operating costs, and improved occupant well-being, setting a new standard for the construction industry.
Real-World Case Studies
Data analysis has been successfully applied in numerous major construction projects worldwide. One notable example is the use of predictive analytics in the construction of the $1.5 billion Mercedes-Benz Stadium in Atlanta, Georgia. By leveraging historical data and machine learning algorithms, the project team was able to accurately forecast potential delays and optimize resource allocation, resulting in the stadium’s completion ahead of schedule.
Another case study involves the implementation of real-time data analytics during the expansion of the Panama Canal. The project team utilized IoT sensors and advanced analytics to monitor equipment performance, track progress, and identify potential issues in real-time. This enabled them to make data-driven decisions, minimize downtime, and ensure the project stayed on track despite its complexity.
The construction of the Hong Kong-Zhuhai-Macau Bridge, one of the world’s longest sea crossings, also benefited from data analytics. The project team employed big data techniques to analyze vast amounts of environmental and structural data collected from sensors embedded in the bridge. This allowed them to monitor the structure’s health, predict maintenance needs, and optimize operations, ensuring the bridge’s long-term performance and safety.
In the United Kingdom, the construction of the Crossrail project, a major railway system in London, relied heavily on data analytics. The project team used advanced analytics to monitor progress, manage risks, and optimize resource allocation across multiple work sites. By integrating data from various sources and applying predictive modeling, they were able to identify potential bottlenecks and take proactive measures to keep the project on schedule and within budget.
These real-world case studies demonstrate the transformative power of data analytics in construction. By harnessing the insights hidden within vast amounts of data, construction professionals can make informed decisions, mitigate risks, and drive better project outcomes. As the industry continues to embrace digital transformation, the adoption of data analytics is set to become increasingly critical for success in the competitive construction landscape.
Challenges and Future Outlook
Data privacy remains a critical concern in the construction industry’s adoption of analytics. Companies must ensure robust data protection measures and adhere to regulations like GDPR when handling sensitive project and employee information. Integrating disparate data sources from various stakeholders, such as architects, contractors, and suppliers, poses another challenge. Developing standardized data formats and interoperable systems is crucial for seamless data exchange and analysis.
Despite these hurdles, the future of data analytics in construction is promising. Advanced technologies like AI, machine learning, and IoT sensors will enable even more sophisticated insights and automation. Predictive analytics will help anticipate and mitigate risks, optimize resource allocation, and improve safety. Building Information Modeling (BIM) and digital twins will facilitate data-driven design and construction processes, reducing errors and enhancing collaboration.
As the industry becomes more data-centric, the role of data analysts and data-savvy professionals will grow in importance. Construction companies that embrace analytics and invest in data-driven decision-making will gain a competitive edge, delivering projects more efficiently and profitably. The future of construction lies in harnessing the power of data to drive innovation, productivity, and sustainability in the built environment.
Conclusion
Data analysis is revolutionizing the construction industry, enabling professionals to make data-driven decisions that enhance project outcomes. By leveraging advanced analytics tools and techniques, construction firms can optimize planning, mitigate risks, boost productivity, and drive innovation in design and materials. Real-world case studies demonstrate the tangible benefits of embracing data analytics, from cost savings to improved safety and quality. While challenges remain, such as data silos and resistance to change, the future of construction lies in harnessing the power of data. As the industry continues to evolve, those who proactively adopt and integrate data analysis into their processes will be well-positioned to thrive in an increasingly competitive landscape.