Smart buildings represent the convergence of artificial intelligence, IoT sensors, and advanced automation systems that are revolutionizing modern facility management. By leveraging smart building metrics and real-time data analytics, these intelligent structures optimize energy consumption, enhance occupant comfort, and reduce operational costs by up to 30%. The integration of AI-driven building management systems (BMS) enables predictive maintenance, automated climate control, and dynamic resource allocation, transforming traditional buildings into responsive, efficient environments that adapt to occupant needs while minimizing environmental impact.
For facility managers and building owners, smart building solutions deliver measurable ROI through reduced energy costs, improved asset longevity, and enhanced operational efficiency. Advanced sensor networks monitor everything from occupancy patterns to equipment performance, providing actionable insights that drive data-informed decision-making. This technological revolution in building management not only addresses immediate operational challenges but also positions properties for long-term sustainability and competitive advantage in an increasingly demanding market.
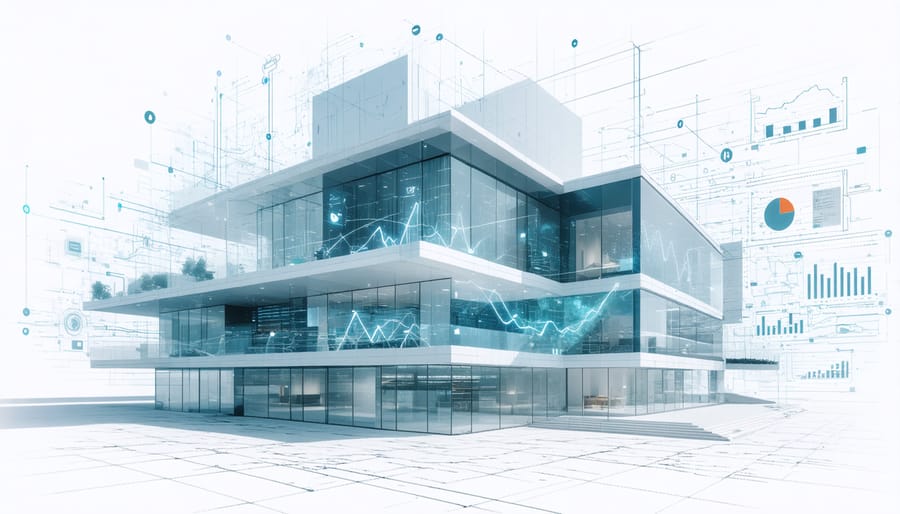
How AI is Revolutionizing Building Energy Management
Real-time Energy Optimization
Advanced AI algorithms are revolutionizing how buildings manage their energy consumption through sophisticated real-time optimization systems. These intelligent systems continuously monitor and analyze multiple data streams from sensors throughout the facility, including occupancy patterns, temperature variations, lighting levels, and equipment performance. By implementing energy-efficient building solutions, facility managers can achieve unprecedented control over their building’s performance.
The AI-driven optimization process operates on three key levels: predictive analysis, real-time adjustment, and continuous learning. Predictive algorithms forecast energy demands based on historical data, weather conditions, and occupancy schedules. Real-time adjustments automatically fine-tune HVAC systems, lighting controls, and other building systems to maintain optimal comfort while minimizing energy waste. The machine learning component continuously refines these operations by identifying patterns and improving efficiency strategies over time.
This dynamic approach typically results in 15-30% energy savings compared to traditional building management systems, while simultaneously improving occupant comfort and reducing maintenance costs. The system’s ability to detect and respond to anomalies also helps prevent equipment failures and extends the lifespan of building systems.
Predictive Maintenance and Performance
Predictive maintenance powered by artificial intelligence represents a paradigm shift in building management, enabling facility managers to address potential issues before they become critical problems. By analyzing real-time data from sensors and building systems, AI algorithms can detect subtle patterns and anomalies that indicate impending equipment failure or performance degradation.
These smart systems continuously monitor key performance indicators such as temperature fluctuations, vibration patterns, and energy consumption rates across HVAC systems, elevators, and other critical infrastructure. Machine learning models process this data to generate accurate maintenance forecasts, allowing building managers to optimize maintenance schedules and reduce costly emergency repairs.
Case studies have shown that AI-driven predictive maintenance can reduce maintenance costs by up to 30% while extending equipment lifespan by 20-25%. For instance, a commercial office complex in Singapore implemented predictive maintenance solutions and reported a 40% reduction in unexpected equipment downtime within the first year of deployment.
The system’s ability to prioritize maintenance tasks based on actual equipment condition, rather than fixed schedules, ensures optimal resource allocation and minimizes unnecessary maintenance interventions. This data-driven approach not only improves operational efficiency but also enhances occupant comfort and satisfaction.
Key Components of AI-Driven Smart Building Systems
Smart Sensors and IoT Infrastructure
Smart sensors and IoT devices form the foundation of modern intelligent buildings, creating a sophisticated digital infrastructure that enables real-time monitoring and control of building systems. These sensor networks typically include occupancy sensors, environmental monitors, energy meters, and equipment performance sensors, all working in concert to collect and transmit critical operational data.
The deployment of these sensors follows a hierarchical architecture, with edge devices collecting data at specific points throughout the building, connected to centralized gateways that aggregate and process information. Advanced sensor systems can monitor multiple parameters simultaneously, including temperature, humidity, CO2 levels, lighting conditions, and space utilization patterns.
Modern IoT sensors incorporate features such as wireless connectivity, extended battery life, and self-diagnostic capabilities, making them both reliable and cost-effective for large-scale deployments. The data collected is transmitted through secure protocols like BACnet, Modbus, or wireless standards such as LoRaWAN, ensuring robust communication while maintaining cybersecurity standards.
Integration platforms consolidate this sensor data into actionable insights, enabling building management systems to make intelligent decisions about resource allocation, maintenance scheduling, and occupant comfort. The scalability of these systems allows for future expansion and adaptation to emerging technologies, making them a foundational element of future-proof building design.
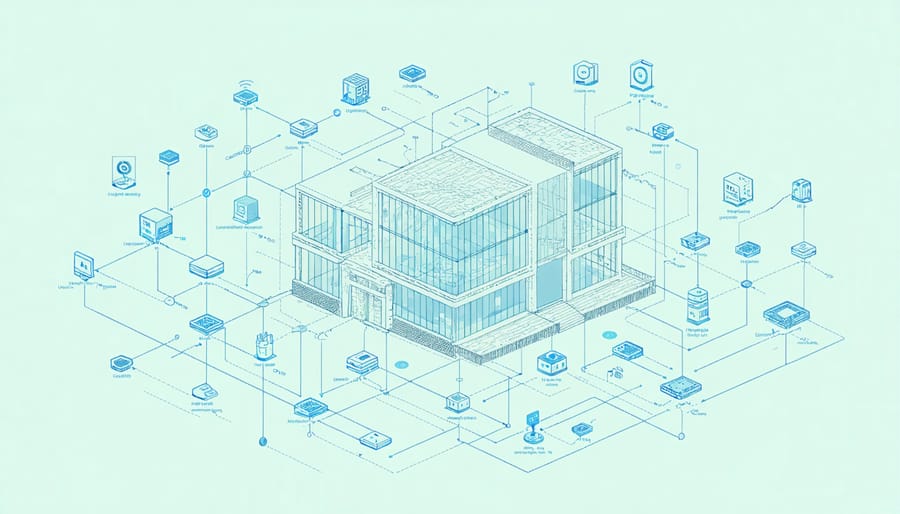
Machine Learning Algorithms and Analytics
Machine learning algorithms form the backbone of modern smart building management systems, leveraging sophisticated predictive analytics to optimize energy consumption and operational efficiency. These AI-driven systems primarily utilize three key algorithmic approaches: supervised learning for pattern recognition in energy usage, reinforcement learning for real-time optimization, and deep learning for complex system behavior analysis.
The supervised learning algorithms analyze historical building data, including occupancy patterns, weather conditions, and energy consumption metrics, to predict future energy demands with remarkable accuracy. These predictions enable proactive adjustments to HVAC systems, lighting, and other building components, typically achieving 15-25% energy savings.
Reinforcement learning algorithms continuously optimize building operations by learning from their actions and their consequences. For instance, these systems can automatically adjust temperature setpoints based on occupancy patterns while maintaining comfort levels, leading to more efficient resource allocation.
Deep learning neural networks process data from multiple building sensors simultaneously, identifying complex correlations that human operators might miss. These networks can detect anomalies in equipment performance, predict maintenance needs, and optimize system operations across multiple variables simultaneously.
Real-time analytics platforms integrate these algorithms with building management systems (BMS) to provide actionable insights. The system processes data from IoT sensors, weather forecasts, and occupancy monitors to make instantaneous adjustments, ensuring optimal performance while maintaining occupant comfort and reducing operational costs.
Implementation Success Stories
Commercial Office Complex Case Study
A recent implementation at the 50-story Hudson Plaza in Chicago demonstrates the transformative impact of smart building solutions on commercial office operations. In 2021, the property management team integrated an AI-powered building management system across their 1.2 million square feet of office space, yielding remarkable results within the first year of deployment.
The system incorporated IoT sensors throughout the building, monitoring occupancy patterns, temperature variations, and equipment performance in real-time. Machine learning algorithms analyzed this data to optimize HVAC operations, lighting controls, and elevator management. The most significant improvements came from predictive maintenance capabilities, which reduced unexpected equipment downtime by 73%.
Key performance metrics from the first 12 months show:
– 32% reduction in overall energy consumption
– $875,000 annual savings in utility costs
– 45% decrease in maintenance-related complaints
– 28% improvement in tenant satisfaction scores
The initial investment of $2.1 million achieved ROI within 2.4 years, exceeding initial projections by 8 months. The building’s energy efficiency rating improved from ENERGY STAR 78 to 92, placing it among the top 10% of commercial office buildings nationwide.
This implementation particularly excelled in demand-response scenarios, automatically adjusting building systems during peak usage periods and contributing to a 41% reduction in peak-hour energy costs.
Healthcare Facility Implementation
St. Mary’s General Hospital in Boston implemented a comprehensive smart building solution in 2021, demonstrating the transformative potential of AI-driven systems in healthcare facilities. The implementation focused on three critical areas: patient comfort optimization, energy efficiency, and operational streamlining.
The facility deployed a network of IoT sensors throughout patient rooms and common areas to monitor temperature, humidity, and air quality in real-time. This system automatically adjusts HVAC settings based on occupancy patterns and patient preferences, resulting in a 23% reduction in energy consumption while maintaining optimal healing environments.
The smart building platform integrates with the hospital’s asset management system, enabling predictive maintenance for critical equipment. AI algorithms analyze data from connected medical devices and building systems, alerting maintenance staff before potential failures occur. This proactive approach has reduced equipment downtime by 47% and decreased emergency maintenance calls by 35%.
Security and access control were enhanced through facial recognition and smart badge systems, ensuring restricted areas remain secure while allowing swift access for authorized personnel during emergencies. The integrated system also monitors patient movement patterns, automatically adjusting lighting and temperature in accordance with daily routines.
Within the first year, the hospital reported an annual energy cost savings of $425,000 and a 30% improvement in patient satisfaction scores related to room comfort and environmental control.
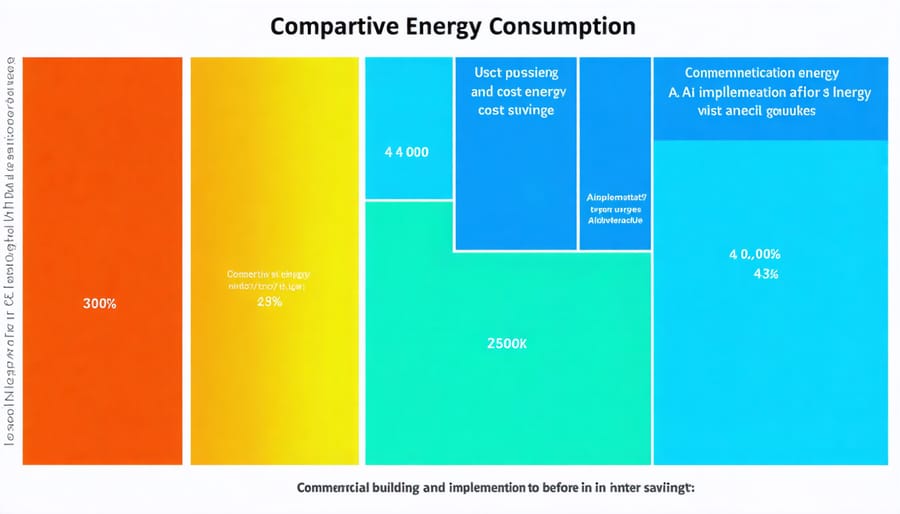
ROI and Financial Benefits
The implementation of smart building solutions demonstrates compelling financial returns through multiple revenue streams and cost reduction opportunities. Recent industry studies indicate that AI-driven building management systems typically achieve a return on investment within 2-4 years, with energy cost savings ranging from 20% to 40% annually.
A comprehensive analysis of 500 commercial buildings revealed that smart HVAC optimization alone resulted in average annual savings of $0.40-$0.60 per square foot. When integrated with intelligent lighting systems and occupancy monitoring, these savings increased to $0.85-$1.25 per square foot annually. These figures align with established sustainability metrics while delivering tangible financial benefits.
Maintenance cost reduction represents another significant ROI driver, with predictive maintenance systems reducing unexpected equipment failures by up to 70% and extending asset life cycles by 20-25%. Labor efficiency improvements through automated monitoring and control systems typically result in 15-20% reduction in facility management staffing costs.
The financial benefits extend beyond direct cost savings. Buildings equipped with smart solutions command premium rental rates, averaging 5-10% higher than comparable traditional properties. Additionally, these buildings experience lower vacancy rates and higher tenant satisfaction scores, contributing to increased property values and better long-term returns.
Insurance providers increasingly offer reduced premiums for smart buildings, recognizing their lower risk profiles due to enhanced monitoring and preventive maintenance capabilities. When combined with government incentives and utility rebates for energy-efficient technologies, these factors significantly enhance the overall financial proposition of smart building investments.
As we look to the future of smart buildings, artificial intelligence stands as the cornerstone of transformation in the construction and facility management industries. The integration of AI-driven solutions has already demonstrated significant improvements in energy efficiency, operational costs, and occupant comfort, but we’re only scratching the surface of its potential. Industry experts predict that by 2030, smart building technologies will become standard practice rather than exceptional features, with AI systems becoming increasingly sophisticated in their ability to predict, adapt, and optimize building performance.
The convergence of IoT sensors, machine learning algorithms, and advanced analytics will continue to push the boundaries of what’s possible in building automation. We’re moving towards truly autonomous buildings that can self-diagnose issues, automatically adjust to changing conditions, and even predict maintenance needs before they become critical problems. This evolution will not only reduce operational costs but also contribute significantly to sustainability goals and carbon reduction targets.
For construction professionals and building owners, staying ahead of these technological advances will be crucial for maintaining competitive advantage and meeting evolving regulatory requirements. The future of smart buildings is not just about technology – it’s about creating more sustainable, efficient, and human-centric spaces that adapt to our needs while minimizing environmental impact.